We use cookies on this website. Cookies help us deliver the best experience on our website. Read about cookies.
-
- Education
- Education
- Programmes and courses
- Applications and admissions
- Tuition fees
- Scholarships
- Exchange studies at Malmö University
- Study Guidance
-
- After admission
- After admission
- Moving to Malmö
- Pre-orientation
- Arrival guide
-
- About studies at Malmö University
- About studies at Malmö University
- Why choose Malmö University
- Understanding university studies
- Connect with our students
On the page -
- Research
- Research
-
- Doctoral studies
- Doctoral studies
- Doctoral courses
-
- Doctoral schools
- Doctoral schools
- Education, Learning and Globalisation
- Doctoral school: Learning in Multicultural Societal Contexts
- ComBine
- Swedish National Graduate School in Science and Technology Education Research
- Doctoral school: Relevancing Mathematics and Science Education (RelMaS)
- Doctoral school: Sustainable Movement Education
- Finding ways in a time of great future challenges (FinnFram)
- Doctoral school: Pedagogy and Vocational Skills
- Doctoral school: Culturally Empowering Education through Language and Literature
- Research subjects
-
- Research centres
- Research centres
- Biofilms Research Centre for Biointerfaces
- Citizen Health
- Imagining and Co-Creating Futures
- Institute for Urban Research
- Malmö Institute for Migration Studies
- Literacy and Inclusive Teaching
- Centre for Work Life Studies
- Sustainable Digitalisation Research Centre
- Centre for Sexology and Sexuality Studies
-
- Research publications
- Research publications
- Search publications
- Malmö University Press
- Research events
- Participate in a research study
- Coffee Break Quiz
On the page -
- Collaboration and Innovation
- Collaboration and Innovation
-
- Areas of collaboration
- Areas of collaboration
- Muvah
- Innovation
- Collaboration with students
-
- Collaborate with researchers
- Collaborate with researchers
- Labs and facilities
- Culture collaboration
- Support Malmö University
- Alumni & Friends
On the page -
- About us
- About us
-
- Faculties and departments
- Faculties and departments
-
- Faculty of Culture and Society
- Faculty of Culture and Society
- Department of Urban Studies
- Department of Global Political Studies
- School of Arts and Communication
-
- Faculty of Education and Society
- Faculty of Education and Society
- Department of Childhood, Education and Society
- Department of Sports Sciences
- Department of Natural Science, Mathematics and Society
- Department of School Development and Leadership
- Department of Culture, Languages and Media
- Department of Society, Culture and Identity
-
- Faculty of Technology and Society
- Faculty of Technology and Society
- Department of Computer Science and Media Technology
- Department of Materials Science and Applied Mathematics
- Faculty of Odontology
- University Dental Clinic
-
- Find and contact Malmö University
- Find and contact Malmö University
- Visit Malmö University
- News and press
- Map of the buildings (Google Maps)
- Merchandise
- Whistleblowing
- Management and decision-making paths
-
- Vision, objectives and strategy 2025
- Vision, objectives and strategy 2025
- Global engagement
- Sustainability
- Widened recruitment and participation
- Quality assurance work at the University
-
- Malmö Academic Choir and Orchestra
- Malmö Academic Choir and Orchestra
- Student work – video pieces
-
- Annual Academic Celebration
- Annual Academic Celebration
- Academic traditions
- Meet our new professors
- The University in a troubled world
On the page
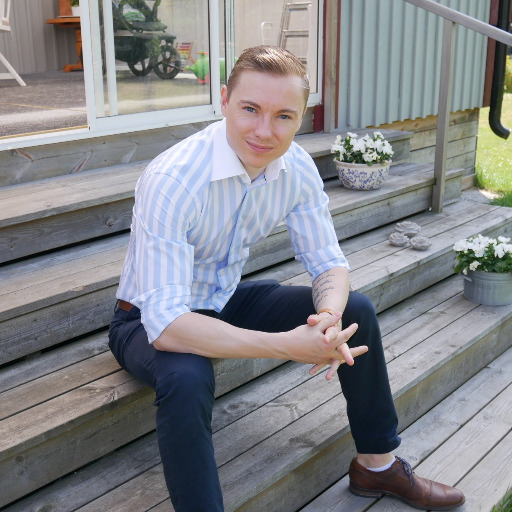
Michael
Belfrage
Doctoral student
michael.belfrage@mau.se
+46 40 665 77 68
orcid.org/0009-0004-1712-5181
Presentation
Michael Belfrage
I am a Computational Social Scientist dedicated to interdisciplinary work which my background reflects, with a BSc in Political Science and an MSc in Computational Social Science. Currently, I am pursuing a WASP-HS PhD in Computer Science at the Faculty of Technology and Society (TS) here at Malmö University where I am also a part of the Internet of Things and People (IoTaP) Research Center. My research primarily focuses on applying computational methods to political science applications. I have a genuine passion for complex systems and Agent-based Models, as well as network science and other graph-theory-related work.
My other responsibilities include holding positions of trust within the university. I represent the PhD-students at the faculty level on the Board for Research and Research Education for TS, and all PhD-students at MAU on the university level through the Doctoral Student Union.
Realizing the Potential of Agent-Based Social Simulation for Public Policy
My research project is a part of the larger overarching WASP-HS project: Realizing the Potential of Agent-Based Social Simulation funded by the Wallenberg Foundations. Together with my supervisors, Paul Davidsson and Fabian Lorig, and my PhD colleague Emil Johansson, we aim to make policy-modelling and Agent-based Simulations a more accessible and reliable tool for policy analysis. Our ambition is to understand how Agent-based Social Simulation (ABSS) – a powerful simulative paradigm capable of imitating human behaviour – and artificial intelligence could be leveraged to facilitate evidence-based policy making in complex social systems. This, we hope to achieve, by identifying policy instruments that can change the causal chains in complex social systems to achieve preferable system-level outcomes. By using ABSS to simulate any complex social system of interest, different 'policy-experiments' can be performed by manipulating these mechanisms in-silico. Empirically evaluating policy options is often infeasible, if not impossible, as it requires a lot of time, funds, and personnel. Without accessible analytical tools to provide insights into the system, decision-makers may have little choice but to engage directly with the target system without any information. Our aim is to allow decision-makers to explore different policy options before implementing them in the real world. By performing these policy-experiments in safe, simulated environments, unintended consequences associated with different policy prescriptions can be identified and diverted.
Publications
-
2025 | Licentiate thesis, comprehensive summary
Agent-based Social Simulation & Policy-Modelling: Facilitating Realistic and Credible Decision-making Support
Michael Belfrage
-
2024 | Article in journal
[In]Credible Models – Verification, Validation & Accreditation of Agent-Based Models to Support Policy-Making
Michael Belfrage, Emil Johansson, Fabian Lorig, Paul Davidsson
-
2024 | Conference paper
Blueprinting Organ Donation: A ‘Policy-first’ Approach for Developing Agent-based Models
Michael Belfrage, Christopher Frantz, Bertilla Fabris, Fabian Lorig
-
2024 | Conference paper
Simulating Change: A Systematic Literature Review of Agent-Based Models for Policy-Making
Michael Belfrage, Fabian Lorig, Paul Davidsson
-
2023 | Report
Uppdragskoncentration i svenska kommuner: Om uppdragsojämlikhet och maktkoncentration
Emanuel Wittberg, Gissur Ó Erlingsson, Michael Belfrage