FACULTY OF CULTURE AND SOCIETY | Seminar
Migration seminar: Modeling Forced Migration with Explainable Machine Learning
Thursday 20 March, 13:15 - 15:00
Niagara, 9th floor, Nordenskiöldsgatan 1, or zoom
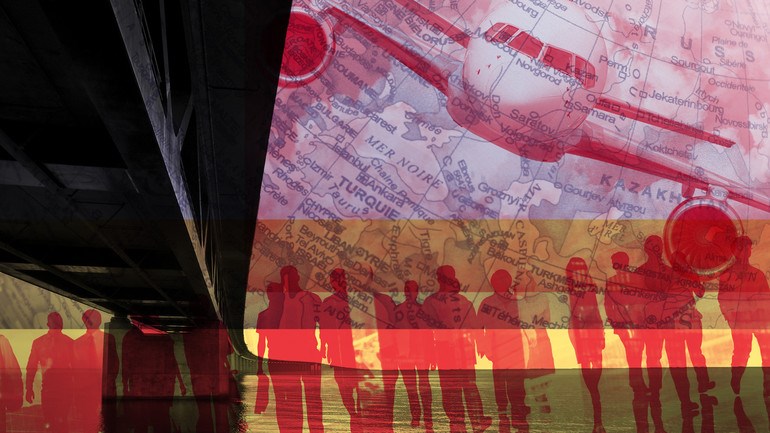
Modeling Forced Migration with Explainable Machine Learning
Welcome to the Migration Seminar!
Profile:
Haodong Qi, Project researcher, Malmö University
Short bio:
Haodong Qi obtained his Ph.D. from Lund University in 2016. He is an economic demographer well-versed in statistics, machine learning, and data science for social research. Currently, Haodong is the principal investigator of the CLIMB project which leverages Big Data and machine learning to better understand and predict Climate-Induced Migration in Africa and Beyond. Since 2023, CLIMB has received generous financial support from the partners of the Belmont Forum: Swedish Research Council, Swedish International Development Cooperation Agency, Scientific and Technological Research Council of Türkiye, Austrian Science Fund, and US National Science Foundation.
Attendance:
This is a hybrid seminar, you are welcome to connect via Zoom or join us at MIM seminar room, floor 9, Niagara, Nordenskiöldsgatan 1. To attend on campus, please gather by the reception area at 13.05.
If you have any questions, send an email mim@mau.se
Zoom
Will be available closer to the seminar date.
Migration models have evolved significantly during the last decade, most notably the so-called flow Fixed-Effects (FE) gravity models. Such models attempt to infer how human mobility may be driven by changing economy, geopolitics, and the environment among other things. They are also increasingly used for migration projections and forecasts. However, recent research showed that this class of models can neither explain, nor predict the temporal dynamics of human movement. This shortcoming is even more apparent in the context of forced migration, in which the processes and drivers tend to be heterogeneous and complex.
In this article, we introduce a novel machine learning model to explain and predict forced migration. Through a case study of Somalis seeking asylum in the EU, we demonstrated three key features of our approach. First, by combining lead-lag analysis and Elastic Net regularization, our model can efficiently extract important predictors and their optimal lags from a high-dimensional space containing thousands of location-specific indicators within a country of origin. This feature is useful for identifying where and when migration responses to climate stressors and socio-economic and political conditions are likely to occur.
Moreover, by training on rolling time windows, our model can analyze the persistence of migration drivers and examine whether persistent drivers might induce qualitative (abrupt) changes in the functioning of migration systems. Finally, compared to common time series extrapolation methods (auto-regressive models), our approach can deliver more accurate forecasts and more reliable assessment of uncertainties. In this regard, our machine learning approach is proven to be not only predictive, but also explainable.