FACULTY OF TECHNOLOGY AND SOCIETY | Dissertation
Dissertation defence – Meenu Mary John
Friday 10 January, 13:15 - 15:15
Niagara, hörsal C, Nordenskiöldsgatan 1, Malmö
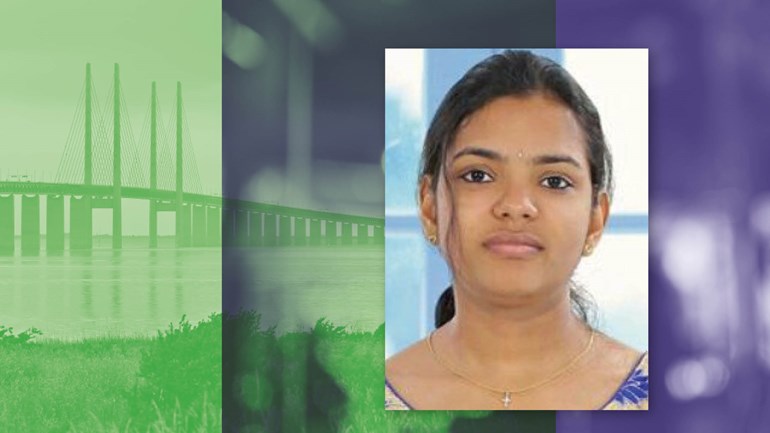
Welcome to Meenu Mary John´s dissertation defence!
Title
Towards Continuous Development of MLOps Practices
Opponent
Dr. Marie Christin Platenius-Mohr, Research Team Manager at ABB
Examining committee
Professor Tommi Mikkonen, University of Jyväskylä
Professor Maria Paasivaara, Lappeenranta-Lahti University of Technology
Associate Professor Stefan Biffl, Vienna University of Technology
Chair at the defence and principal supervisor
Professor Helena Holmström Olsson, Malmö University
Other supervisors
Professor Jan Bosch, Chalmers
About the research
With digitalisation, companies that specialise in software-intensive embedded systems are experiencing a transformation from a business reliant on hardware and products to a business that utilises software, data and AI (especially Machine Learning (ML) and Deep Learning (DL)).
These technologies allow companies to extend their product offerings, develop new products or services, and position themselves as industry leaders by creating new revenue opportunities. Despite the promising advancements in high-performance parallel hardware and the growing interest in software, data and AI, the majority of ML/DL deployments fail within companies. This highlight the need to optimise the end-to-end process of developing, deploying, and evolving ML/DL models. Also, this demands a strong understanding and collaboration between researchers and practitioners in both the fields of ML/DL and Software Engineering to gain a holistic perspective on engineering ML systems into software-intensive systems.
The research focuses on two main themes: (a) Establishing systematic and structured frameworks for the development, deployment and evolution of models, and (b) Exploring how the evolving (changing) needs of software-intensive embedded systems companies utilising ML/DL technologies shape the MLOps (Machine Learning Operationalisation) practises they needed and measures its maturity over time. Based on the themes mentioned above, we have three primary objectives: (a) Identifying the need for MLOps, (b) Developing the frameworks for MLOps adoption, and (c) Standardising and measuring the MLOps practices.
The thesis shows well-structured approach to improve end-toend ML lifecycle. Through the research, we seek to enable and advance not only experts but also non-experts to effectively approach the development, deployment, and evolution of ML/DL models in the current embedded systems industry. This is relevant considering the shortage of highly skilled data scientists.